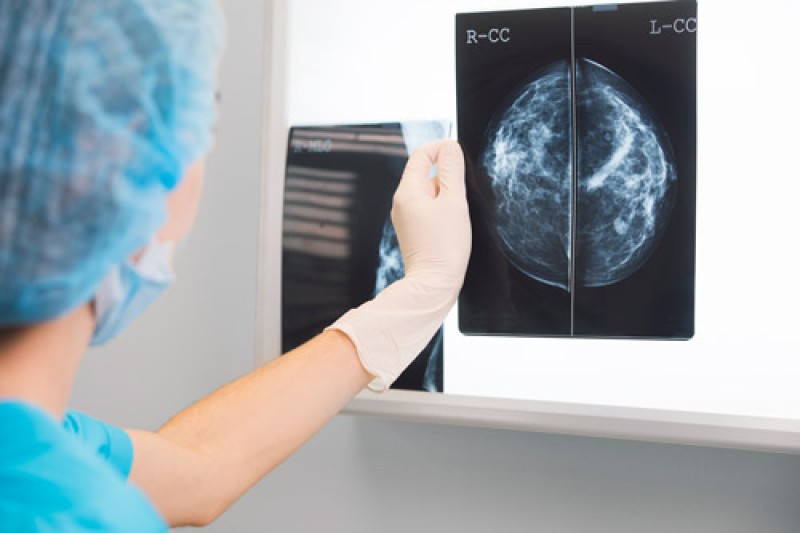
New research from Bournemouth University has implemented an effective new way to turn data about breast cancer tumours into features that can help machine learning models predict whether they are malignant or benign.
The results showed that of the six machine learning models tested, the “decision tree” model could predict a tumour’s status with an accuracy of over 98%.
Breast cancer is the most commonly diagnosed cancer in the world. A report by the World Health Organization (WHO) stated that there were 685,000 deaths related to breast cancer and 2.3 million women were diagnosed with breast cancer in 2020.
Early diagnosis is crucial in improving survival rates. In recent years machine learning techniques have shown the potential to play a significant role in speeding up a patient’s diagnosis, alongside the main clinical methods of biopsy, mammography, and physical examination.
In this new study, published in the journal BioMedInformatics, researchers introduced an effective feature engineering approach to extract features from data relating to 569 breast cancer tumours contained in the Wisconsin Breast Cancer Diagnosis Dataset.
The engineered features were then used on six well-known machine-learning models:
- Random Forest
- Logistic Regression
- Decision Tree
- K-Nearest neighbour
- Multi-layer Perceptron
- Extreme Gradient Boosting
The results highlighted the Decision Tree model's superior performance, achieving an impressive average accuracy of 98.64%. Random Forest was the next most successful with 97%, whilst K-Nearest Neighbour had the lowest accuracy with 89%.
A decision tree model works in a similar way to a flowchart - guiding the model through a sequence of decisions based on a feature’s values, ultimately leading to a classification or prediction.
Each feature of a tumour is considered as a branching point. Starting from the top, the model evaluates the feature and progresses down the tree through a series of branches, each representing a possible value range for that feature. This process continues until the model reaches a final prediction about whether the tumour is malignant or benign, based on the cumulative decisions made along the branches.
“These findings could have significant benefits both for the scientific community looking develop new ways in which AI can help speed up diagnosis of breast cancer, and also for the medical community when deciding on the best treatment for their patients,” explained Emilija Strelcenia, Postgraduate Researcher at Bournemouth University, who led the study.
"While our study focused on a single dataset, the methodology we employed for analysing tumour characteristics holds promise for broader application with diverse datasets. Moreover, there is potential to explore a variety of machine learning models beyond the six we utilized, further enhancing the efficiency of breast cancer detection," she suggested.